Helper or Hinderer? Will AI Replace or Reinforce Stack Overflow's Digital Community?
Can AI replicate this vibrant community's wisdom, or is the unique human element irreplaceable?
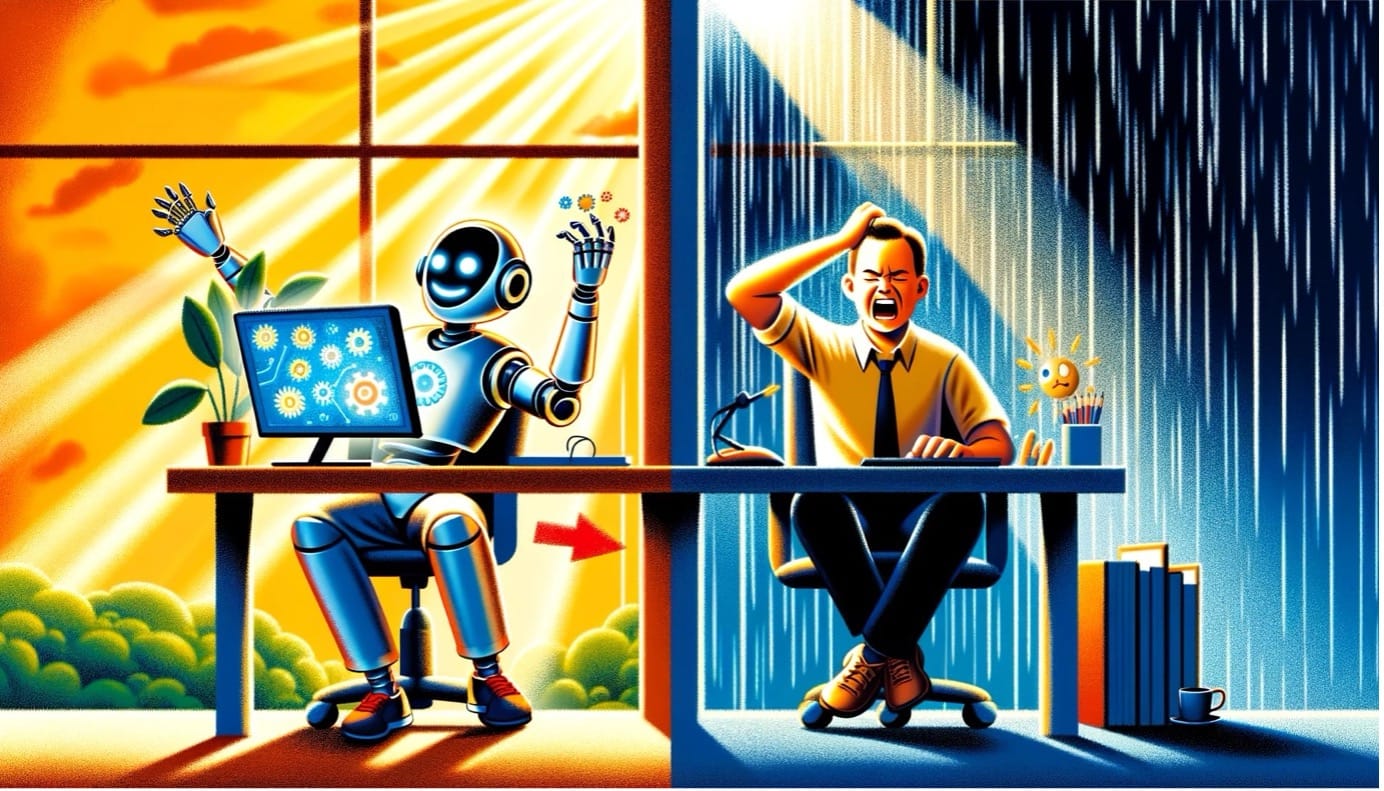
For the past decade, Stack Overflow has been a developer's best friend. Its undeniable utility and vast knowledge base act as a beacon of collective knowledge. But as AI's shadow looms, a pressing question emerges: Can AI replicate this vibrant community's wisdom, or is the unique human element irreplaceable?
Stack Overflow, a popular Q&A platform for programmers, fosters collaborative expertise through its voting system, capturing programming trends and skills (Vasilescu & Filkov, 2013; Moutidis & Williams, 2021). However, generative AI's impact on code generation and debugging raises questions about embracing AI-assisted development or upholding peer-reviewed human expertise. How Stack Overflow navigates this crossroads could redefine its core purpose as a focal point for developers and a barometer for technological shifts shaping software development's future.
The proposed analysis model merges the Technology Acceptance Model (TAM) with the Sociotechnical Systems Theory and the Critical Theory of Technology, counteracting TAM's neglect of social elements and the interplay of relationships (Venkatesh & Davis, 2000; Bagozzi, 2007). This approach examines the interplay between individual and societal interactions (Orlikowski & Iacono, 2001) and how power structures in technology are formed (Feenberg, 1991). By merging TAM's individual-centric approach with these broader perspectives, the framework aims to ensure that AI developments enhance, rather than destabilise, community-based knowledge networks.
Figure 1 presents the proposed framework.
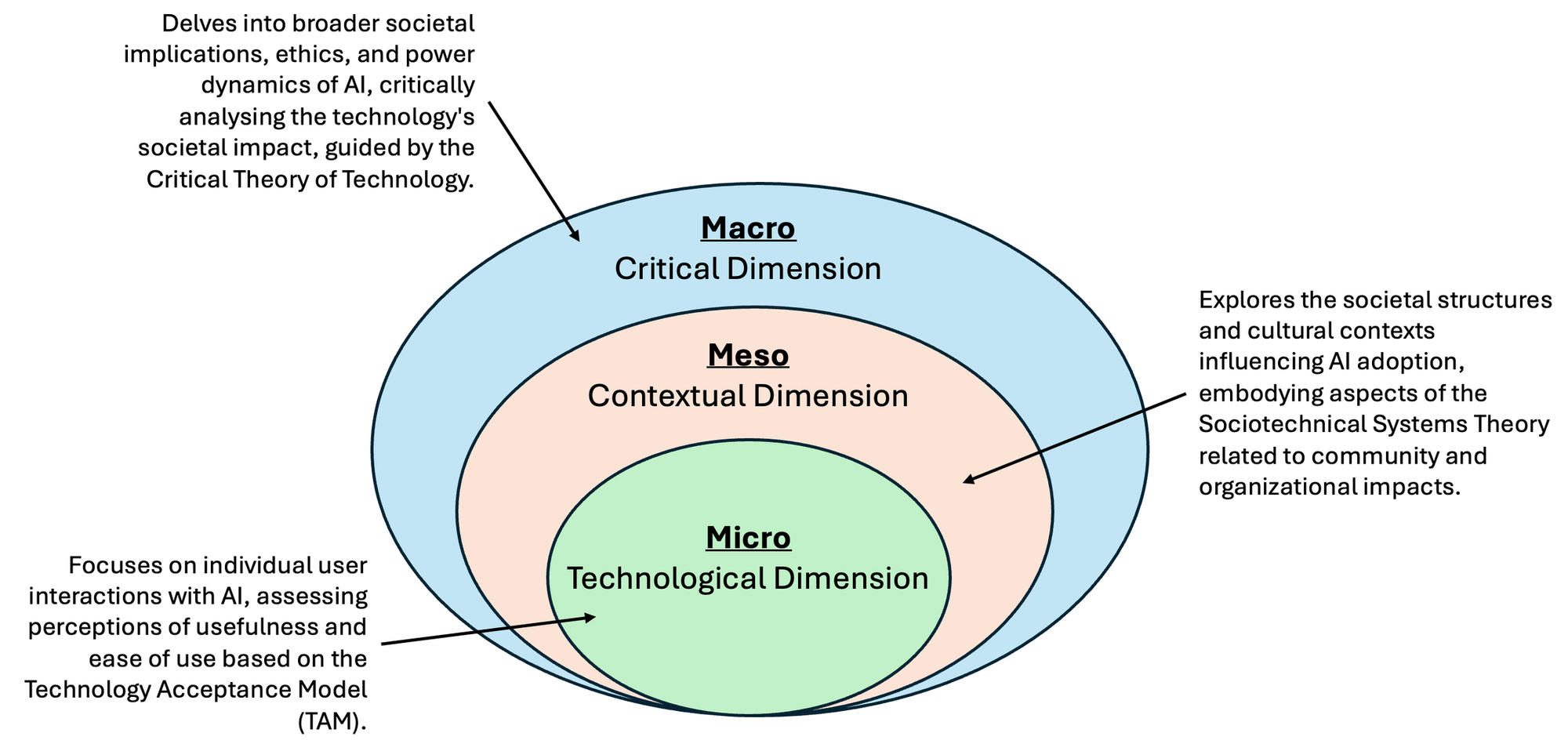
TAM Analysis
Exploring the intersection of humans and AI, this analysis zeroes in on AI tools' perceived effectiveness and user-friendliness. The goal? To uncover why Stack Overflow users might adopt or resist AI integration.
Figures 2 and 3 present the TAM (Davis, 1989) and TAM analysis for Stack Overflow users.
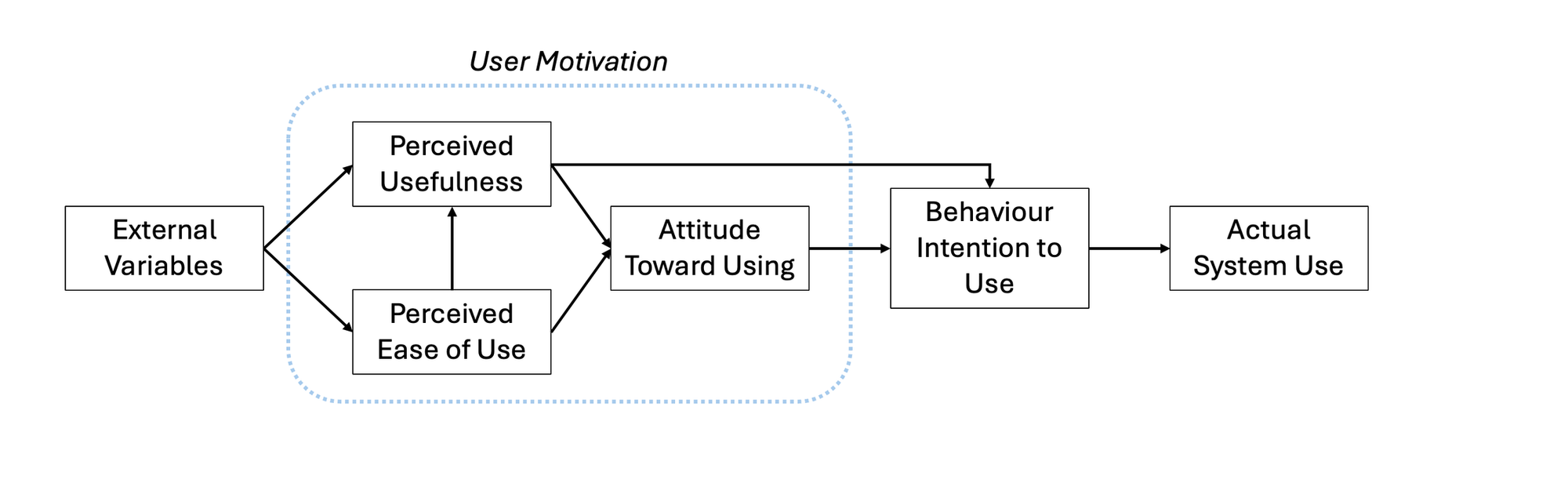
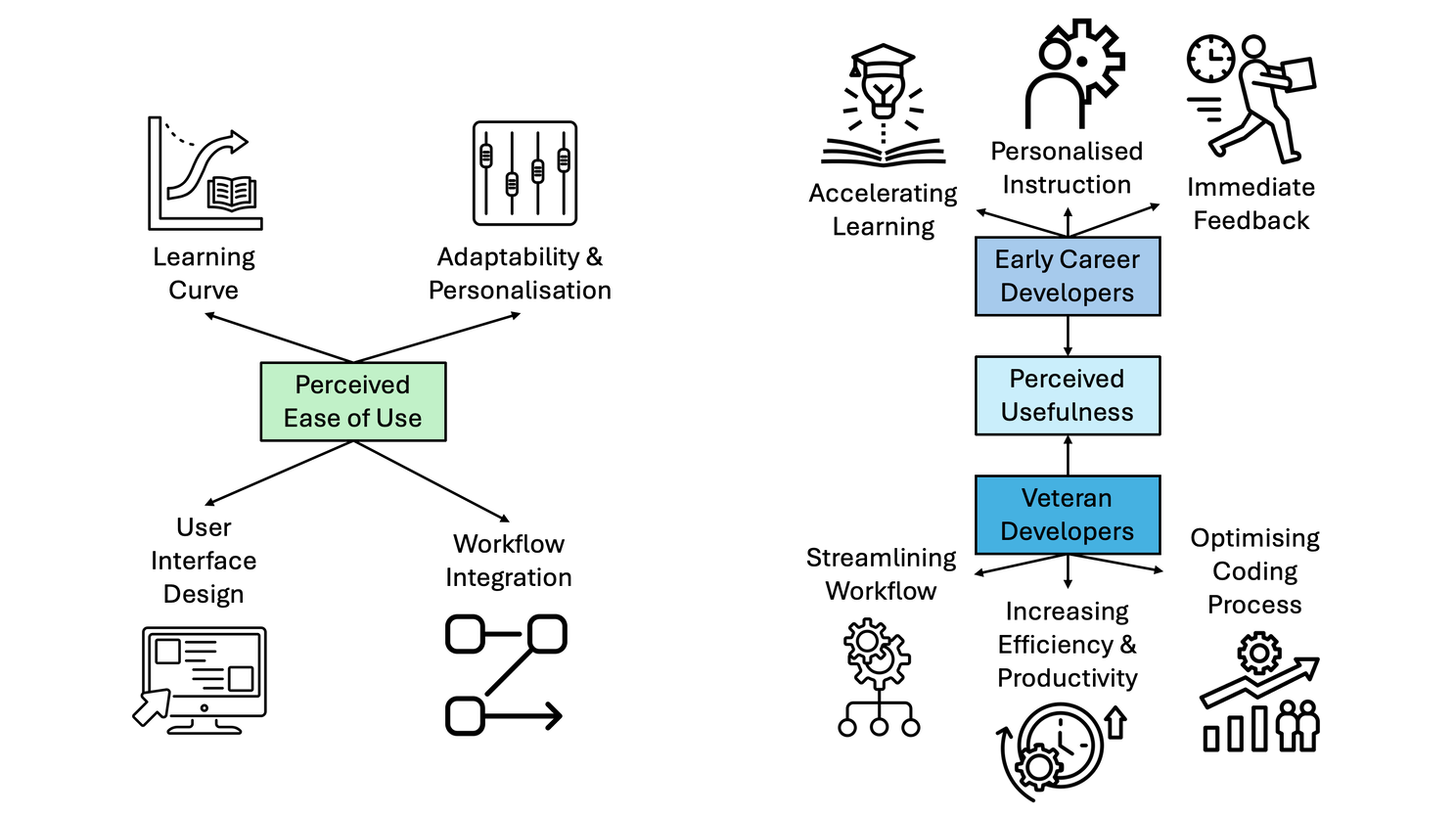
Perceived Ease of Use
When introducing new AI tools, the learning curve, influenced by user expertise and support availability, affects perceived ease of use and widespread adoption (Amershi et al., 2019). Integrating AI tools into developer workflows through user-friendly interfaces has reduced this learning curve, which aligns with successful UX design principles (Shneiderman & Plaisant, 2010). However, the subjective nature of "natural workflows" means imposing predetermined interfaces could constrain creativity and problem-solving abilities. Code suggestions within editors could disrupt pre-existing thought processes and hinder codebase comprehension.
Furthermore, if the perceived ease of AI tools inadvertently undermines developer autonomy, it could erode the essential problem-solving and coding skills foundational to software development. Therefore, adaptable AI tools augmenting developer productivity across iterations without compromising quality are necessary, not just preferable. Achieving lasting adoption requires a deep understanding of human factors shaping workflows, enabling enhancements that genuinely empower and include developers.
Perceived Usefulness
While AI assistants help reduce keystrokes and speed up tasks (Liang et al., 2024), their perceived benefit, as cited by veterans for workflow efficiency, like productivity increases of over 55% (Peng et al., n.d.) and by novices for learning support (Sun et al., 2024), masks a more profound concern: the trade-off between convenience and skill development.
With reports of developers turning to AI for brainstorming yet shunning it for final coding due to hallucinated outputs (Das et al., 2024), it's evident that over-reliance on AI could stunt the growth of a developer's expertise. The push for AI-driven learning and productivity raises concerns about undervaluing the depth of skill gained through manual coding, risking a future where developers are more operators than craftspeople.
Upon reflection, balance is critical, leveraging AI's benefits while safeguarding the irreplaceable human touch. This strategy ensures that AI tools enhance the developer's skill, not eclipse, with judgment guiding their deployment. Fusing AI with human skill creates a complementary, not competitive, coding landscape.
Sociotechnical System Theory Analysis
Unpacking AI's influence on Stack Overflow calls for viewing the platform as a tapestry of social and technical strands—a perspective grounded in Sociotechnical Systems Theory (Trist & Bamforth, 1951). It highlights AI's interplay with Stack Overflow's social fabric, emphasising that technology integration is inseparable from existing culture and communal interplay.
Figure 4 presents the meso analysis for Stack Overflow.
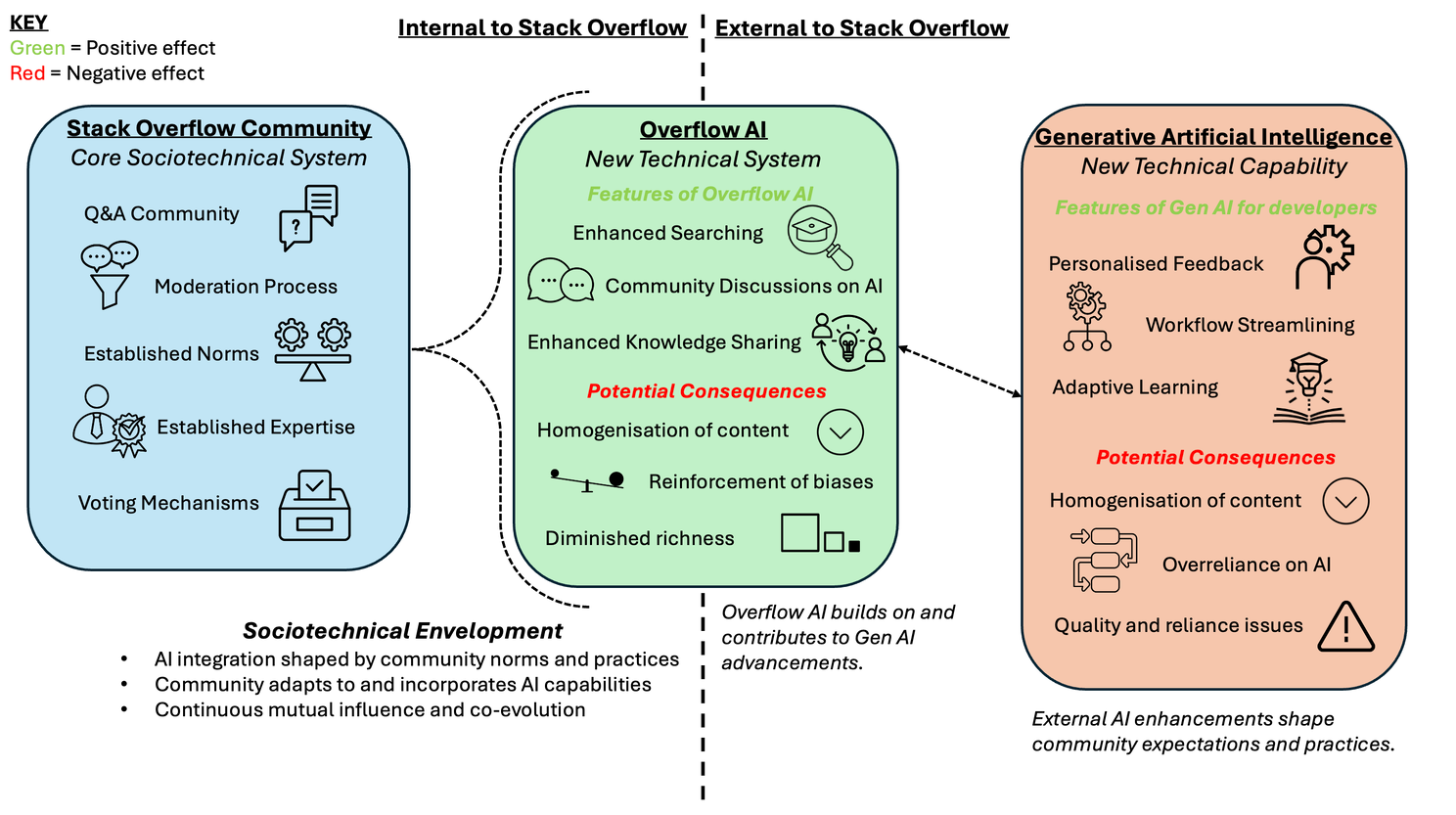
Stack Overflow initially banned AI-generated content in 2022 due to quality concerns. Research by Kabir et al. (2024) showed that 52% of ChatGPT answers contained inaccuracies, and 77% were verbose. However, 35% of participants still preferred AI outputs for comprehensiveness, highlighting a potential gap between Stack Overflow's policies and some users' perceived needs for AI-assisted knowledge delivery. In hindsight, the outright ban oversimplified complex sociotechnical dynamics, overlooking AI's potential evolution and opportunities for human-AI collaboration to augment community expertise through effective integration (Asatiani et al., 2021). This indicates a possible disconnect between Stack Overflow's quality control policies and a segment of its user base's perceived needs.
A year later, Stack Overflow introduced Overflow AI, enhancing search, fostering AI discussions, and streamlining knowledge sharing. This collaborative workspace aims to unlock new avenues for problem-solving through the mutual enhancement of individual intelligence (Levy, 2015), signalling a shift towards embracing AI while harmonising it with community norms and the existing social and technical fabric.
Overflow AI combines AI's efficiency with Stack Overflow's reliability, optimising the sociotechnical knowledge-sharing system for collective intelligence (Aurigemma & Panko, 2012; Arazy et al., 2016). This aligns with the view of online communities as sociotechnical systems where technology and social processes intertwine (Arazy et al., 2016). However, introducing AI may elicit unintended social responses undermining existing norms and power dynamics (Faraj et al., 2011), necessitating proactive cultivation of a new sociotechnical equilibrium through sociotechnical envelopment.
Moreover, Overflow AI’s introduction risks homogenising answers, diminishing nuance from human diversity and entrenching existing biases (Candelon et al., 2023). This content homogenisation stifles creativity and may entrench existing biases, as AI systems often learn from data sets that may not represent diverse perspectives. Consequently, this could undermine Stack Overflow's core strength – diverse community perspectives.
Stack Overflow's evolution hinges on striking a harmonious balance between human ingenuity and AI efficiency. It can fortify its community's wisdom by championing diversity, scrutinising AI contributions, and promoting transparency. Failure risks diluting its knowledge-sharing prowess.
Critical Theory of Technology Analysis
Through the lens of Critical Theory (Feenberg, 1991), Gen AI emerges as a potent social actor within Stack Overflow, potentially reshuffling the developer hierarchy. As AI automates tasks and opens doors to advanced data analysis and broader coding access (Davenport & Ronanki, 2018), it challenges traditional power dynamics, potentially side-lining human expertise. Will this disruption pave the way for a more democratic and accessible future for coding education?
Figure 5 presents the critical actors of Stack Overflow.
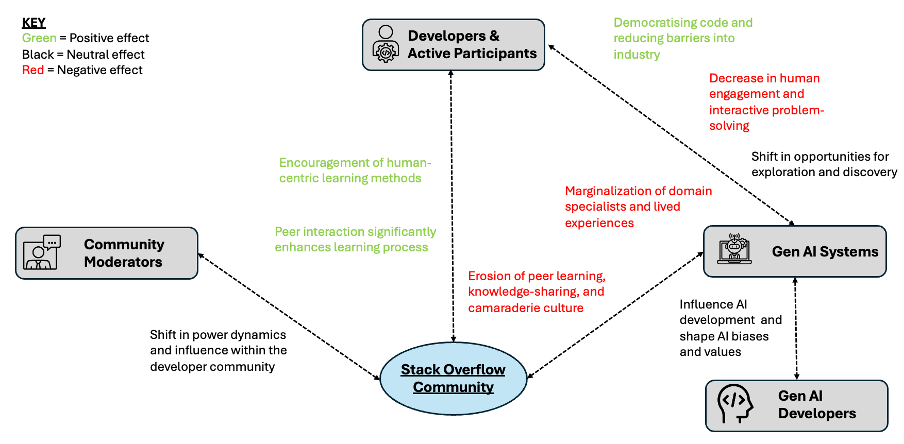
While AI's societal potential to democratise coding knowledge, offering wider access and streamlining tedious tasks is clear, this paints an incomplete picture. It is clear that AI can perform these functions, but how does this reshape developer society?
Historically, human developers and moderators wielded dominant control over content curation and quality through voting systems (Shaw & Hill, 2014). However, introducing Gen AI systems like Overflow AI threatens to upend this power dynamic. These AI models inherently reflect the biases and values of their creators, potentially marginalising specific methodologies based on flawed underlying data and algorithms (Gutiérrez, 2023).
Moreover, by lowering coding barriers and displacing human experts as authoritative sources, AI risks marginalising domain specialists and their nuanced expertise (Seo et al., 2021). An over-reliance on AI solutions could critically undermine core community dynamics - the very peer interactions, idea exchanges, and collaborative feedback loops that have fostered rich learning and educational depth (An et al., 2022). Reducing these elements diminishes Stack Overflow's foundation of collective intelligence cultivated through discourse.
The rise of AI-driven knowledge dissemination threatens Stack Overflow's socio-cultural foundations. Traditional methods, like mentorship and hands-on coding, foster deep understanding and problem-solving (Robins et al., 2003), which is crucial in the developer community.
Therefore, if AI systems become the primary source for accessing and consuming coding knowledge, it could fundamentally reshape how developers learn, collaborate and view expertise within their community. An overreliance on AI-generated content might erode the culture of peer learning, knowledge-sharing, and camaraderie that has characterised software development practices.
The developer society faces a pivotal shift as Stack Overflow folds Gen AI into its ecosystem. The potential to democratise learning and enhance collaboration is undeniable. However, this must be navigated without dimming the spark of human-driven mentorship and cooperation central to coding's culture. The challenge lies in leveraging AI to amplify, not overshadow, the developer's human touch.
Conclusion and Recommendations
Introducing Gen AI into Stack Overflow's community presents opportunities and challenges. While AI can enhance knowledge sharing, streamline workflows, and lower entry barriers, it also risks homogenising content, altering balanced power dynamics and eroding the richness of peer learning. Stack Overflow must balance AI's benefits while maintaining the community's foundational values.
How can Stack Overflow embrace AI while safeguarding its community's values?
Figure 6 outlines recommendations for AI's future integration.
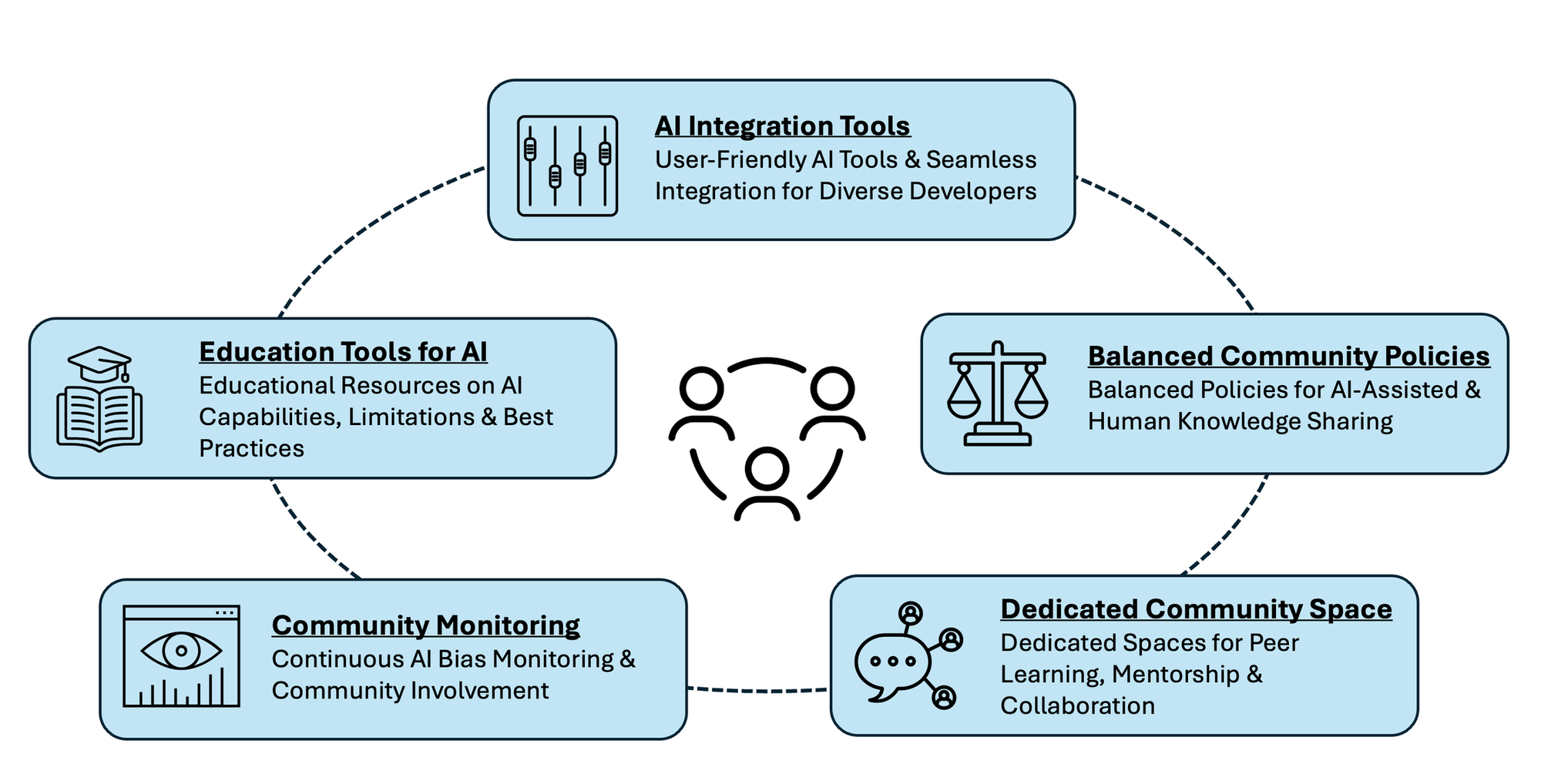
References
Amershi, S., Weld, D., Vorvoreanu, M., Fourney, A., Nushi, B., Collisson, P. & Teevan, J. (2019). Guidelines for human-AI interaction. In Proceedings of the 2019 CHI Conference on Human Factors in Computing Systems (pp. 1-13).
An, X., Hong, J-C., Li, Y. & Zhou, Y. (2022). The impact of attitude toward peer interaction on middle school students' problem-solving self-efficacy during the COVID-19 pandemic. In Frontiers in Psychology, Available at: https://doi.org/10.3389/fpsyg.2022.978144. [Accessed 12th April 2024]
Arazy, O., Daxenberger, J., Lifshitz-Assaf, H., Nov, O., & Gurevych, I. (2016). Turbulent stability of emergent roles: The dualistic nature of self-organizing knowledge coproduction. Information Systems Research, 27(4), 792-812.
Asatiani, A., Malo, P., Nagbøl, P. R., Penttinen, E., Rinta-Kahila, T., & Salovaara, A. (2021). Sociotechnical envelopment of artificial intelligence: An approach to organizational deployment of inscrutable artificial intelligence systems. Journal of the Association for Information Systems, 22(2), 325-352.
Aurigemma, S. D., & Panko, T. (2012). Socio-Technical Systems Theory and Work System Design. Available at: https://www.researchgate.net/publication/258822123_Socio-Technical_Systems_Theory_and_Work_System_Design [Accessed 17th April 2024]
Bagozzi, R. P. (2007). The legacy of the technology acceptance model and a proposal for a paradigm shift. Journal of the association for information systems.
Ballen, C. J., Aguiar, A., Awatramani, M., Boly, A., Farmer, A., Gan, X., & Lee, S. (2019). Uncovering bios through coded narrative data: A research note on computational data translation practices. Population Research and Policy Review, 38(6), 801-828.
Bench-Capon, T.J.M. & Dunne, P.E. (2007). Argumentation in artificial intelligence. Artificial Intelligence, Available at: https://www.sciencedirect.com/science/article/pii/S0004370207000793 [Accessed 8th April 2024]
Candelon, F., Krayer, L., Rajendran, S., & Martínez, D. Z. (2023). How People Can Create—and Destroy—Value with Generative AI. Available at: https://www.bcg.com/publications/2023/how-people-create-and-destroy-value-with-gen-ai [Accessed 4th April 2024]
Das, J. K., Mondal, S., & Roy, C. K. (2024). Investigating the Utility of ChatGPT in the Issue Tracking System: An Exploratory Study. Available at: https://arxiv.org/abs/2402.03735 [Accessed 29th March 2024]
Davenport, T.H. & Ronanki, R. (2018). Artificial Intelligence for the Real World. Harvard Business Review, 96(1), 108-116.
Davis, F. D. (1989). Perceived usefulness, perceived ease of use, and user acceptance of information technology. MIS Quarterly, 13(3), 319-340.
Dégallier-Rochat, S., Kurpicz-Briki, M., Endrissat, N., & Yatsenko, O. (2022). Human augmentation, not replacement: A research agenda for AI and robotics in the industry. Front. Robot. AI, 9:997386. doi: 10.3389/frobt.2022.997386 [Accessed 24th March 2024]
Driscoll J. (1994) Reflective practice for practise. Senior Nurse. Vol.13 Jan/Feb. 47 -50
Faraj, S., Jarvenpaa, S.L. & Majchrzak, A. (2011). Knowledge collaboration in online communities. Organization Science, 22(5), 1224-1239.
Feenberg, A. (1991). Critical Theory of Technology. Oxford University Press.
Gutiérrez, J. L. M. (2023). On actor network theory and algorithms: ChatGPT and the new power relationships in the age of AI. AI and Ethics. doi: 10.1007/s43681-023-00314-4
Hasanein, A. M., & Sobaih, A. E. E. (2023). Drivers and Consequences of ChatGPT Use in Higher Education: Key Stakeholder Perspectives. Eur J Investig Health Psychol Educ, 13(11), 2599-2614. doi: 10.3390/ejihpe13110181
Ibitoye, O., Simaki, V., & Stol, K. J. (2021). Evidence for discrimination against Africans in the open source communities. 2021 IEEE/ACM 43rd International Conference on Software Engineering: Software Engineering in Practice (ICSE-SEIP) (pp. 211-220). IEEE.
Islam, S., Bhuiyan, M., Huq, S., & Usman, M. (2021). Auditing machine learning algorithms for bias: A scoping review. arXiv preprint arXiv:2112.08394.
Kabir, S., Udo-Imeh, D.N., Kou, B. & Zhang, T. (2024). Is Stack Overflow Obsolete? An Empirical Study of the Characteristics of ChatGPT Answers to Stack Overflow Questions. In Proceedings of the CHI Conference on Human Factors in Computing Systems (CHI '24), May 11-16, Honolulu, HI, USA. ACM, New York, NY, USA. Available at: https://doi.org/10.1145/3613904.3642596 [Accessed 15th April 2024]
Lévy, P. (2015). The Age of Collective Intelligence. New York: Basic Books.
Liang, J.T., Yang, C. & Myers, B.A. (2024). A Large-Scale Survey on the Usability of AI Programming Assistants: Successes and Challenges. Proceedings of the IEEE/ACM 46th International Conference on Software Engineering (ICSE '24), Association for Computing Machinery, New York, NY, USA, Article 52, pp. 1-13. Available at: https://doi.org/10.1145/3597503.3608128 [Accessed 4th April 2024]
Maleki, N., Padmanabhan, B., & Dutta, K. (2024). AI Hallucinations: A Misnomer Worth Clarifying. Available at: https://arxiv.org/pdf/2401.06796 [Accessed 4th April 2024]
Moutidis, I. & Williams, H.T.P. (2021). Community evolution on Stack Overflow. PLOS ONE, 16(6), e0253010. Available at: https://doi.org/10.1371/journal.pone.0253010 [Accessed 5th April 2024]
Open AI ChatGPT (2024) Blog Image
Orlikowski, W. J., & Iacono, C. S. (2001). Research commentary: Desperately seeking the "IT" in IT research—A call to theorizing the IT artifact. Information Systems Research, 12(2), 121-134.
Patro, G. K., Chakraborty, S., Sarker, I. H., & Majumder, M. (2022). Machine learning for identifying code clones without metadata: Challenges, state-of-the-art, and future directions. arXiv preprint arXiv:2201.06754.
Peng, S., Kalliamvakou, E., Cihon, P. & Demirer, M. (n.d.). The Impact of AI on Developer Productivity: Evidence from GitHub Copilot. Available at: https://ar5iv.labs.arxiv.org/html/2302.06590 [Accessed 12th April 2024]
Robins, A., Rountree, J., & Rountree, N. (2003). Learning and Teaching Programming: A Review and Discussion. Computer Science Education, 13(2), 137-172.
Seo, K., Tang, J., Roll, I., Fels, S. & Yoon, D. (2021). The impact of artificial intelligence on learner–instructor interaction in online learning. In International Journal of Educational Technology in Higher Education, 18, Article 54. Available at: https://doi.org/10.1186/s41239-021-00292-9 [Accessed 17th April 2024]
Shaw, A., & Hill, B. M. (2014). Laboratories of Oligarchy? How the Iron Law Extends to Peer Production. Journal of Communication, 64(2), 215-238.
Shneiderman, B., & Plaisant, C. (2010). Designing the user interface: Strategies for effective human-computer interaction (5th ed.). Addison-Wesley.
Sun, D., Boudouaia, A., Zhu, C. et al. (2024). Would ChatGPT-facilitated programming mode impact college students’ programming behaviors, performances, and perceptions? An empirical study. International Journal of Educational Technology in Higher Education Available at: https://doi.org/10.1186/s41239-024-00446-5. [Accessed 4th April 2024]
Trist, E. L., & Bamforth, K. W. (1951). Some Social and Psychological Consequences of the Longwall Method of Coal-Getting. Human Relations, 4(1), 3-38.
Vasilescu, B., Filkov, V. & Serebrenik, A. (2013). StackOverflow and GitHub: Associations Between Software Development and Crowdsourced Knowledge. In Proceedings - SocialCom/PASSAT/BigData/EconCom/BioMedCom 2013, pp. 188-195. doi: 10.1109/SocialCom.2013.35
Venkatesh, V., & Davis, F. D. (2000). A theoretical extension of the technology acceptance model: Four longitudinal field studies. Management science, 46(2), 186-204.
Zhuang, Y., Wu, F., Chen, C., et al. (2017). Challenges and opportunities: from big data to knowledge in AI 2.0. Frontiers Inf Technol Electronic Eng, 18, 3–14. doi: 10.1631/FITEE.1601883